Voxel data analysis – development of algorithms for segmentation and crack detection of computed tomography data | Funding Period 2
In the project, algorithms are being developed for the automatic analysis of computed tomography data with the aim of determining material properties, recognizing the position and orientation of carbon reinforcements in the matrix, and detecting (micro-)cracks. Special challenges in voxel data segmentation arise due to the low density differences of the investigated materials. Accordingly, powerful neural network based voxel data segmentation methods are developed. An additional focus lies on the development of 4D methods for segmentation in time series of computed tomography data in dynamic experiments.
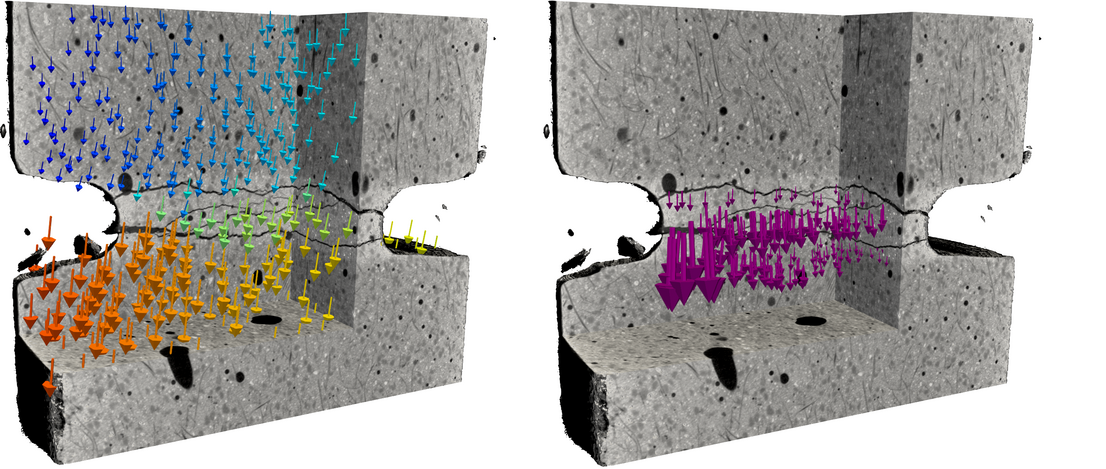
Scientists
![Prof. Dr. sc. techn. habil. Hans-Gerd Maas [Translate to English:] Hans-Gerd Maas](/fileadmin/_processed_/6/1/csm_Maas_Quadratisch_616a498495.jpg)
D-01062 Dresden (Germany)
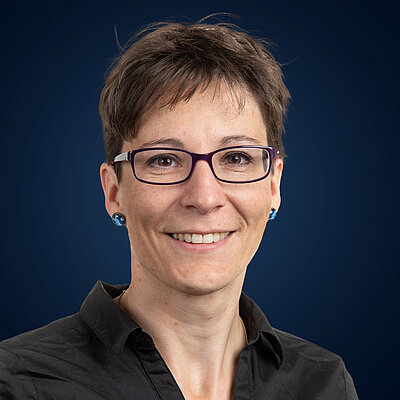
01062 Dresden (Germany)
![M.Sc. Bindusara Nagathihalli Lokesh [Translate to English:]](/fileadmin/_processed_/0/2/csm_platzhalter_232740995a.jpg)
01062 Dresden (Germany)
Cooperations
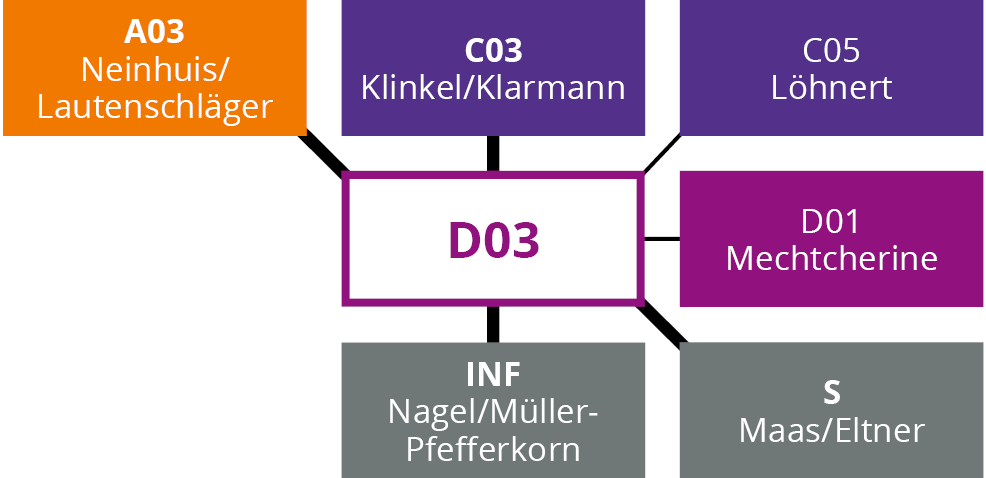
Ehemalige / Former involved
Noch nix hier, da keine Veränderung im Abgang
Development of Advanced Tomography Data Analysis Techniques | Funding Period 1
In project D03, the focus is on the development of extended methods for tomography data analysis. The approach is to investigate the components of the specimens by segmenting them subvoxel-precisely and predict the distribution of the contained carbon fibers.
After the development of appropriate algorithms, the samples will be deformed by the application of force and then scanned again in the tomograph. The goal then is to determine the relationship between structure and stability. The multitemporal data sets produced in this process are matched using 3D-LSM (least squares matching) in order to investigate not only the displacement vectors (caused by the temporal difference) but also the deformations and cracks with subvoxel accuracy. With the aim to further optimize the data quality and thus the data analysis, a sensor modeling and corresponding calibration strategies are developed, which largely eliminate the systematic errors of tomographs.
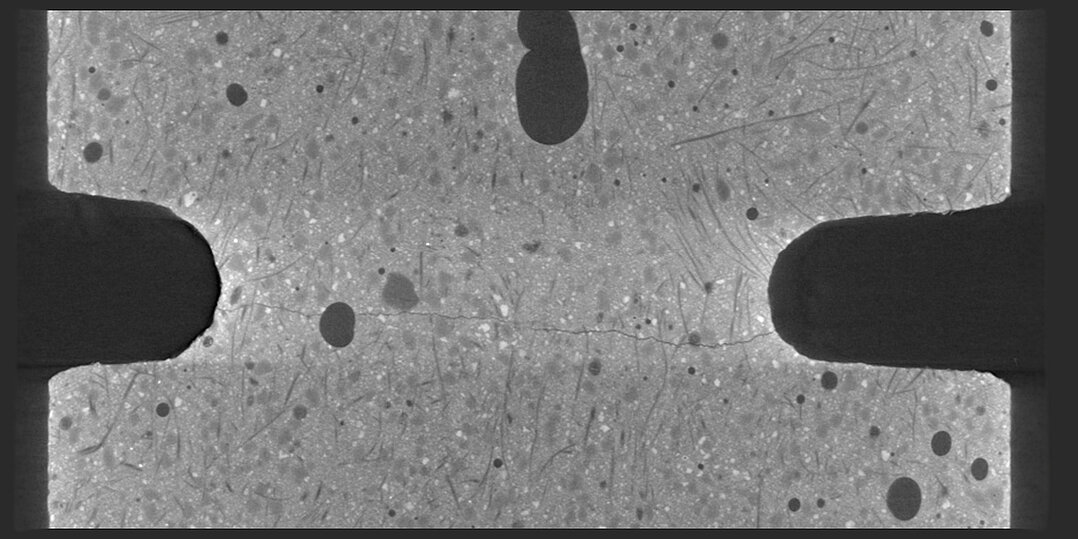
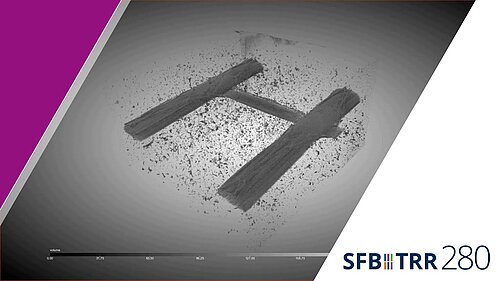
Publikationen | Publications
Giese, J.; Herbers, M.; Liebold, F.; Wagner, F.; Grzesiak, S.; de Sousa, C.; Pahn, M.; Maas, H.-G.; Marx, S.; Curbach, M.; Beckmann, B. (2023) Investigation of the Crack Behavior of CRC Using 4D Computed Tomography, Photogrammetry, and Fiber Optic Sensing in Buildings 13, issue 10, 2595 – DOI: https://doi.org/10.3390/buildings13102595
Hardner, M.; Liebold, F.; Wagner, F.; Maas, H.-G. (2024) Investigations into the Geometric Calibration and Systematic Effects of a Micro-CT System in: Sensors 24, issue 16, 5139 – DOI: https://doi.org/10.3390/s24165139
Liebold, F.; Lorenzoni, R.; Curosu, I.; Léonard, F.; Mechtcherine, V.; Paciornik, S.; Maas, H.-G. (2021) 3D Least Squares Matching Applied to Micro-Tomography Data in: The International Archives of the Photogrammetry, Remote Sensing and Spatial Information Sciences 43, issue B2, p. 533–539 – DOI: 10.5194/isprs-archives-XLIII-B2-2021-533-2021
Liebold, F.; Maas, H.-G. (2022) 3D-Deformationsanalyse und Rissdetektion in multitemporalen Voxeldaten von Röntgentomographen in: Kersten, T.; Tilly, N. [eds.] Proc. der 42. Wissenschaftlich-Technischen Jahrestagung der DGPF, Band 30, 05./06.10.2022 in Dresden, p. 105–116 – DOI: 10.24407/KXP:1796026123
Liebold, F.; Wagner, F.; Giese, J.; Grzesiak, S.; de Sousa, C.; Beckmann, B.; Pahn, M.; Marx, S.; Curbach, M.; Maas, H.-G. (2023) Damage Analysis and Quality Control of Carbon-Reinforced Concrete Beams Based on In Situ Computed Tomography Tests in Buildings 13, issue 10, 2669 – DOI: https://doi.org/10.3390/buildings13102669
Mester, L.; Klempt, V.; Wagner, F.; Scheerer, S.; Klarmann, S.; Vakaliuk, I.; Curbach, M.; Maas, H.-G.; Löhnert, S.; Klinkel, S. (2023) A Comparison of Multiscale Methods for the Modelling of Carbon-Reinforced Concrete Structures in: Ilki, A.; Çavunt, D.; Çavunt, Y. S. [eds.] Building for the Future: Durable, Sustainable, Resilient – Proc. of fib Symposium 2023, 05.–07.06.2023 in Istanbul (Turkey), publ. in: Lecture Notes in Civil Engineering 350, Cham: Springer, p. 1418–1427 – DOI: 10.1007/978-3-031-32511-3_145
Mester, L.; Wagner, F.; Liebold, F.; Klarmann, S.; Maas, H.-G.; Klinkel, S. (2022) Image-based modelling of carbon-fibre reinforced concrete shell structures in: Stokkeland, S.; Braarud, H. C. [eds.] Concrete Innovation for Sustainability – Proc. for the 6th fib International Congress 2022, 12.–16.06.2022 in Oslo (Norway), Oslo: Novus Press, p. 1631–1640.
Vakaliuk, I.; Scheerer, S.; Liebold, F.; Wagner, F.; Kruppa, H.; Vollpracht, A.; Curbach, M. (2024) Properties of the High-Performance Matrix of TRC Elements Cast Under Vacuum Conditions in: Mechtcherine, V.; Signorini, C.; Junger, D. [eds.]: Transforming Construction: Advances in Fiber Reinforced Concrete – Proc. of XI RILEM-fib Int. Symp. on Fiber Reinforced Concrete (BEFIB 2024), 15.–18.09.2024 in Dresden, publ. in RILEM Bookseries, Vol. 54, Cham: Springer Nature Switzerland, p. 786–793 – https://doi.org/10.1007/978-3-031-70145-0_93
Wagner, F.; Eltner, A.; Maas, H.-G. (2023) River water segmentation in surveillance camera images: A comparative study of offline and online augmentation using 32 CNNs in: International Journal of Applied Earth Observation and Geoinformation 119, 103305 – DOI: 10.1016/j.jag.2023.103305
Wagner, F.; Maas, H.-G. (2023) A Comparative Study of Deep Architectures for Voxel Segmentation in Volume Images in: The International Archives of the Photogrammetry, Remote Sensing and Spatial Information Sciences XLVIII-1/W2-2023, p. 1667–1676 – https://doi.org/10.5194/isprs-archives-XLVIII-1-W2-2023-1667-2023
Wagner, F.; Mester, L.; Klinkel, S.; Maas, H.-G. (2023) Analysis of Thin Carbon Reinforced Concrete Structures through Microtomography and Machine Learning in: Buildings 13, issue 9, 2399 – DOI: 10.3390/buildings13092399
Dissertation | Doctoral thesis
Franz Wagner: Segmentation in Tomography Data: Exploring Data Augmentation for Supervised and Unsupervised Voxel Classification with Neural Networks [Doktorarbeit | doctoral thesis]. TU Dresden, Datum der mündlichen Prüfung | Date of oral examination: 12.07.2024, publiziert | published: 23.09.2024, https://nbn-resolving.org/urn:nbn:de:bsz:14-qucosa2-936719
Datensätze | Data sets
Blanch, X.; Wagner, F.; Eltner, A. (2023) River Water Segmentation Dataset (RIWA) at: Kaggle – DOI: 10.34740/KAGGLE/DSV/4901781
Wagner, F. (2023) Carbon Rovings Segmentation Dataset (RIWA) at: Kaggle – DOI: 10.34740/KAGGLE/DS/2920892
Wagner, F. (2023) Concrete Pores Segmentation Dataset (RIWA) at: Kaggle – DOI: 10.34740/KAGGLE/DS/2921245
Wagner, F. (2023) Fiber Segmentation Dataset (RIWA) at: Kaggle – DOI: 10.34740/KAGGLE/DS/2894881